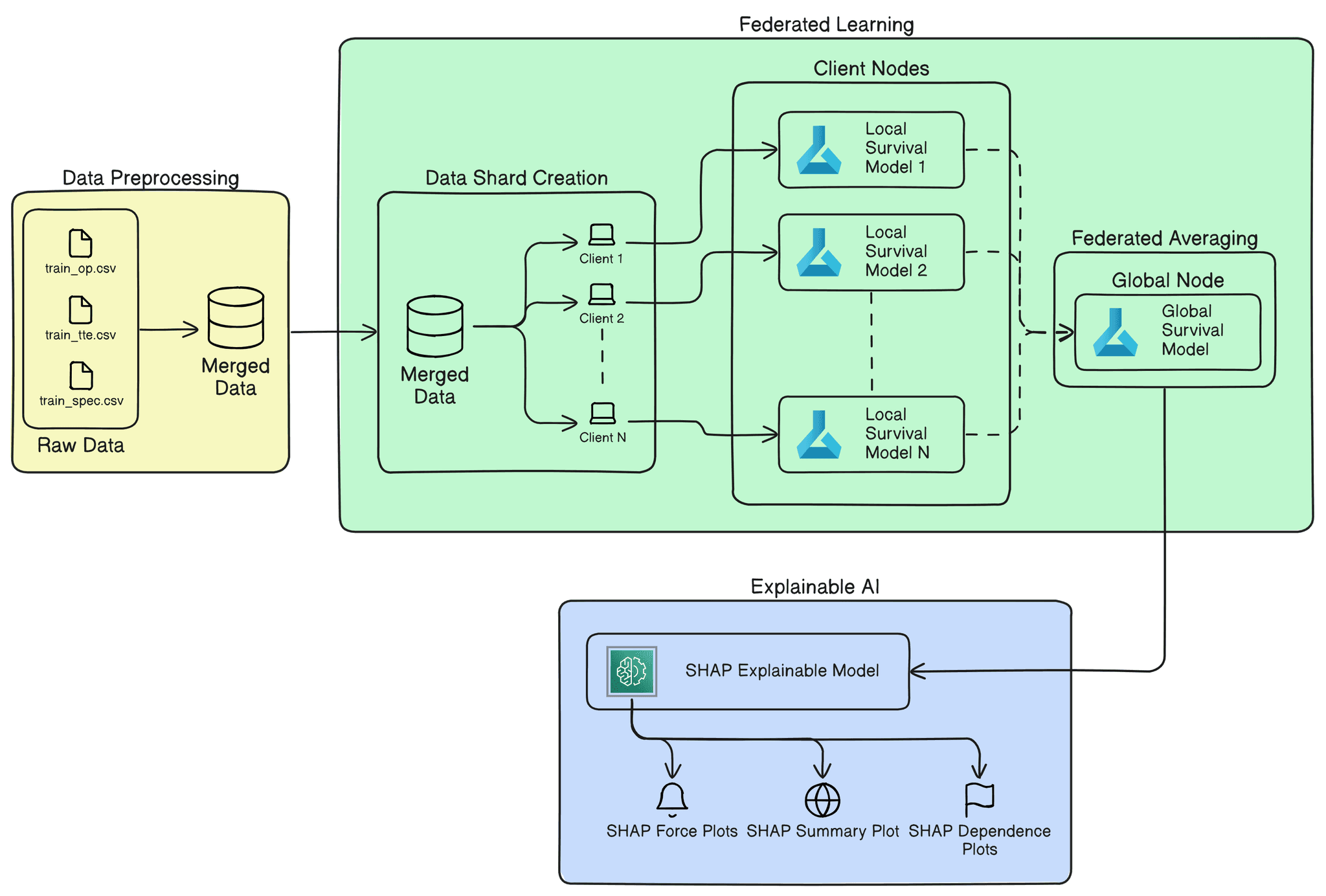
Predictive Maintenance in SCANIA Trucks
Mitigating failures by using Federated Learning and Explainable AI
Predictive maintenance is a vital approach in modern industries, enabling reduced downtime, optimized resources, and enhanced operational efficiency. This study develops a federated survival analysis framework augmented with explainable AI (XAI) to predict the Remaining Useful Life (RUL) of components and assess failure risks while preserving data privacy.
The framework leverages federated learning to aggregate insights from decentralized data across multiple clients. Three survival models—Random Survival Forest (RSF), Gradient Boosting Survival Analysis (GBSA), and Support Survival Vector Machines (SSVM)—were evaluated across 10 federated clients over 10 rounds. Model performance was assessed using the Concordance Index (C-index), where RSF outperformed the others with global training and testing C-index values of 0.7577 and 0.7376, respectively. GBSA followed closely, while SSVM exhibited comparatively lower accuracy, highlighting its limitations in federated environments.
Explainability was achieved through SHAP (SHapley Additive exPlanations), providing insights into feature contributions to model predictions. Key features, including 666_0, 309_0, and 158_8, were identified as critical predictors of survival outcomes. SHAP dependence plots illustrated complex feature interactions, while instance-specific force plots highlighted individual component risks, empowering maintenance teams to target high-risk components proactively.
The RSF survivability plot demonstrated declining survival probabilities over time, enabling predictive maintenance planning. By identifying components with steeper survival declines, planners can prioritize repairs or replacements, reducing costs and operational disruptions. The federated approach successfully handled client heterogeneity, synthesizing insights from diverse data distributions without compromising data privacy.