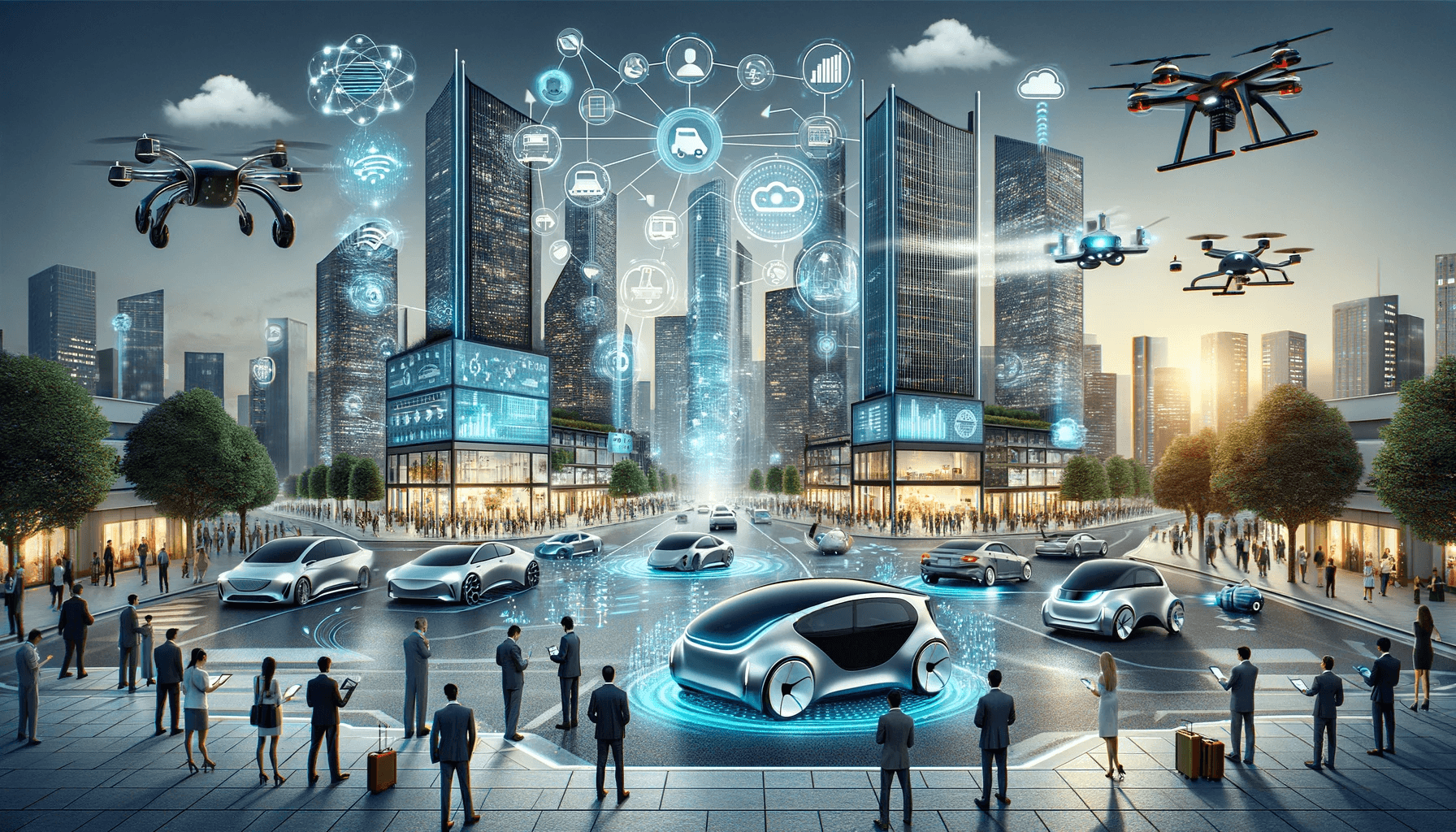
FL for State of Charge Prediction
Data-Driven SoC Forecasting in Electric Vehicles: A Federated Learning Perspective
Electric Vehicles (EVs) are pivotal for sustainable transportation, but their widespread adoption is hindered by challenges in accurately estimating the State of Charge (SoC). Current SoC estimation methods often lack precision, leading to range anxiety among EV users. This study explores Federated Learning to enhance SoC estimation in EVs. This approach promises more accurate and privacy-conscious predictions, aiming to improve EV reliability and user confidence, and support sustainable mobility. This study is motivated by this transformative shift in India's mobility sector and seeks to contribute to this movement by enhancing SoC estimation in EVs, supporting the government's vision of a cleaner, more efficient, and technologically advanced transportation system.
Model Performance Consistency: Across the rounds of federated learning, there was a consistent performance in SoC prediction accuracy. The R-squared (R2) values were predominantly high, suggesting that a significant portion of the variance in SoC was captured by the models.
Error Metrics Trends: The Mean Squared Error (MSE), Mean Absolute Error (MAE), and Root Mean Squared Error (RMSE) metrics fluctuated across rounds, indicating the models' varying degrees of prediction precision in different rounds. The general trend for these error metrics decreased, suggesting that the model was adjusting well to the SoC prediction task over time.
Optimization Over Rounds: The optimization of models as evidenced by the error metrics and R2 value typically showed improvement as the number of rounds increased. This indicates that the federated learning process is effectively refining the model's predictive capabilities with each round.