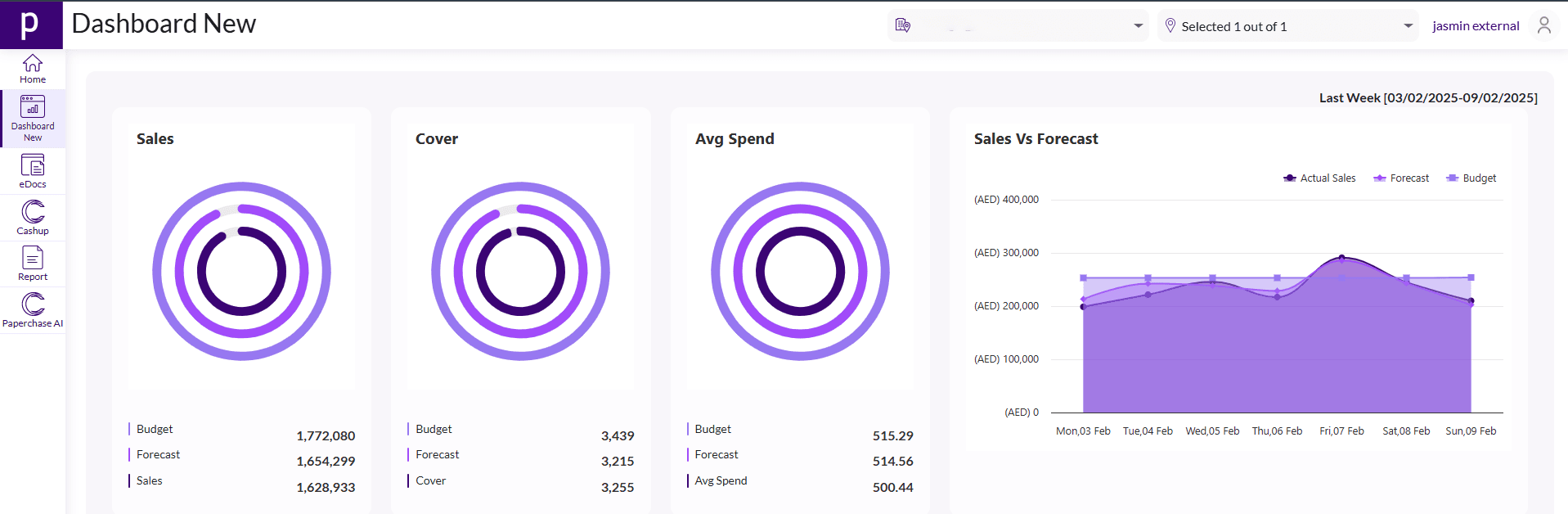
Advanced Time Series Forecasting
Time Series Forecasting with Exogenous Variables for Restarurants Globally
This project explores the application of various forecasting models to predict sales data in a real-world business environment, focusing on both traditional time series models and advanced machine learning techniques. The study aims to compare the performance of models such as SARIMA and Exponential Smoothing with cutting-edge machine learning models, including Long Short-Term Memory (LSTM), NBEATS, and NHITS. Additionally, the research investigates the utility of automated machine learning platforms like Azure AutoML and AutoTS in simplifying the forecasting process while maintaining accuracy.
The comparative analysis reveals that while traditional models are effective for simpler time series data with strong seasonal components, they struggle with complex patterns and are less adaptable to changes in data trends. In contrast, machine learning models, particularly LSTM and NBEATS, demonstrate superior performance in capturing intricate patterns and long-term dependencies, offering more accurate forecasts. Automated machine learning platforms significantly reduce the time required for model selection and optimization, making them accessible for businesses with limited data science expertise.
The models were deployed in a production environment, where AutoTS was used for daily sales forecasting and Azure AutoML for hourly predictions. The results showed that both models delivered accurate forecasts closely aligned with actual sales data, with Mean Absolute Percentage Errors (MAPE) of 7% for daily forecasts and 7.67% for hourly forecasts. These findings underscore the practical utility of advanced forecasting models in business operations, providing actionable insights for decision-making in areas such as inventory management and staffing.
The project concludes that while traditional models have their place, the integration of machine learning and automated tools into forecasting practices offers substantial advantages in terms of accuracy, adaptability, and efficiency. Future research directions include the integration of external data sources, real-time model retraining, the development of hybrid models, and the exploration of these methodologies across different industries. This work lays a strong foundation for the continued enhancement of forecasting models, with the potential to significantly impact business decision-making processes.